Edge Detection
Giacomo Boracchi
Contents
- Building filters as smoothing + differentiating filters
- Differentiating Filters
- Compute image Principal Derivative (i.e. directional derivatives along axis)
- Hard Threshold - Play on the choice of the Threshold T
- morphological operations on the mask
- compute edges using Canny method (it includes nonmax suppression and histeresis thresholding)
close all
clear
clc
Building filters as smoothing + differentiating filters
disp('differentiating filters') diffx=[1 -1] diffy = diffx' %smoothing filters Previtt sx=ones(2,3); sy=sx'; % build Previtt derivative filters disp('derivative filters Previtt') dx=conv2(sy , diffx) dy=conv2(sx , diffy) %smoothing filters Sobel sx=[1 2 1 ; 1 2 1]; sy=sx'; % Build Sobel derivative filters disp(' derivative filters Sobel') dx=conv2(sy,diffx) dy=conv2(sx,diffy)
differentiating filters diffx = 1 -1 diffy = 1 -1 derivative filters Previtt dx = 1 0 -1 1 0 -1 1 0 -1 dy = 1 1 1 0 0 0 -1 -1 -1 derivative filters Sobel dx = 1 0 -1 2 0 -2 1 0 -1 dy = 1 2 1 0 0 0 -1 -2 -1
Differentiating Filters
y = im2double(imread('image_lena512.png')); figure(1), imshow(y),title('original image'); % compute gradient components (horizontal and vertical derivatives) Gx=conv2(y , dx , 'same'); Gy=conv2(y , dy , 'same'); figure(3),imshow(Gx, []),title('horizontal derivative') figure(4),imshow(Gy, []),title('vertical derivative') % Gradient Norm M=sqrt(Gx.^2 + Gy.^2); figure(5),imshow(M,[]),title('gradient magnitude')
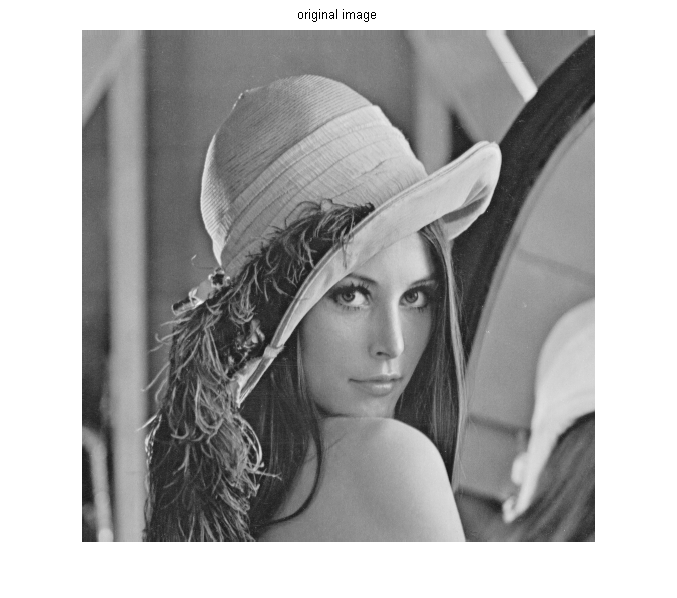
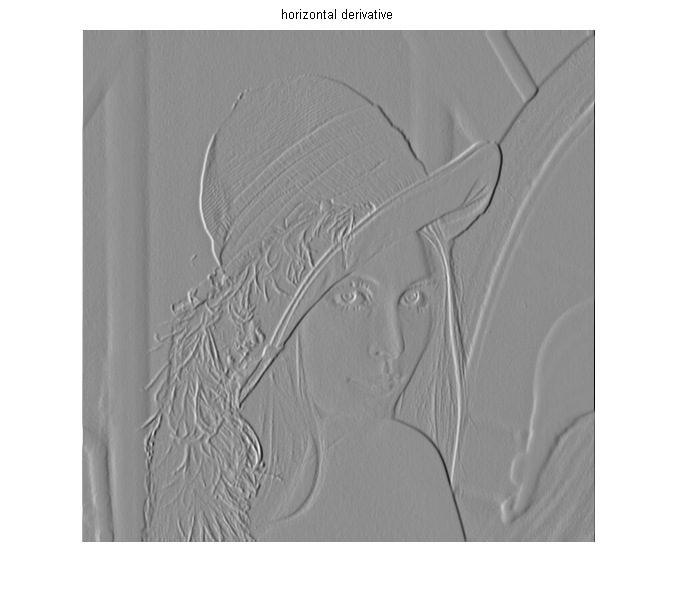
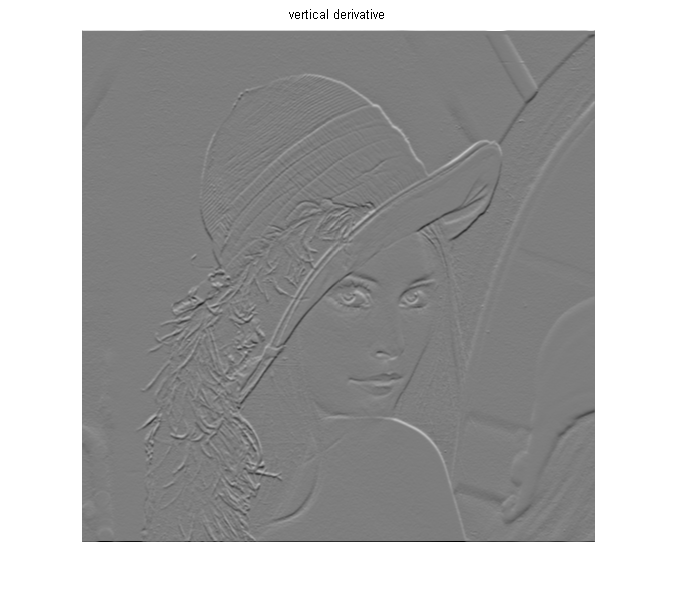
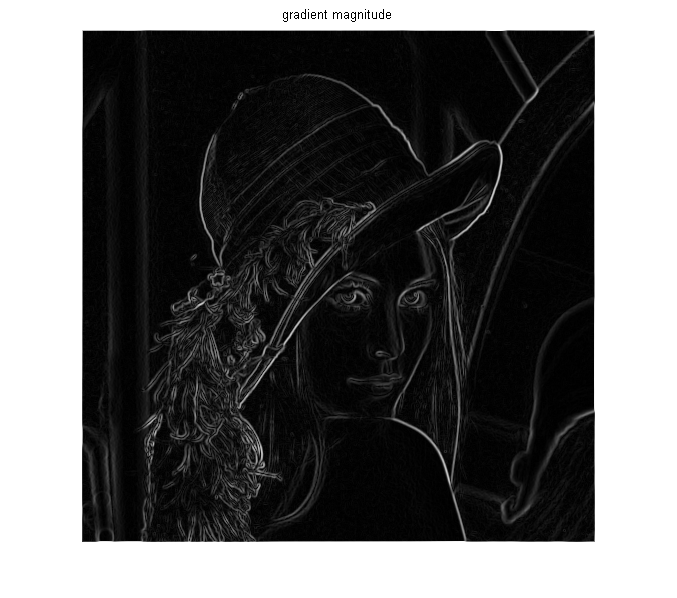
Compute image Principal Derivative (i.e. directional derivatives along axis)
% build the gradient image as a two plane image: the first plane contains horizontal derivatives, the second plane the % vertical ones Grad=zeros(size(y,1),size(y,2),2); Grad(:,:,1)=Gx; Grad(:,:,2)=Gy; % compute Gradient norm Norm_Grad = sqrt(Grad(: , : , 1) .^ 2 + Grad(: , : , 2) .^ 2); BORDER = 3; % remove boundaries as these are affected by zero padding Norm_Grad(1 : BORDER, :) = 0; Norm_Grad(end - BORDER : end, :) = 0; Norm_Grad(:, 1 : BORDER) = 0; Norm_Grad(:, end - BORDER : end) = 0; % we change the sign of the derivative because the y axis is "increasing downwards", since in Matlab it correspodns to the row index Dir_Grad=atand(- sign(Grad(:,:,1)).*Grad(:,:,2) ./(abs(Grad(:,:,1))+eps)); figure(2),imshow(Norm_Grad,[]),title('norm'); figure(3),imshow(Dir_Grad,[]),title('directions');
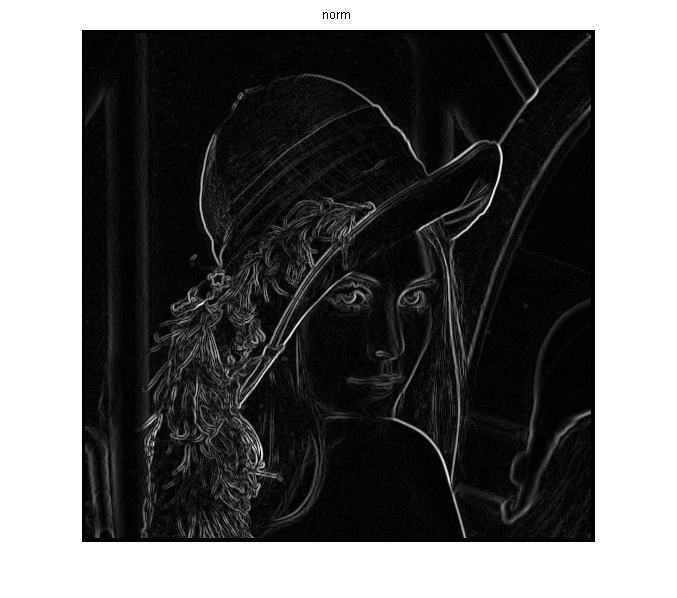
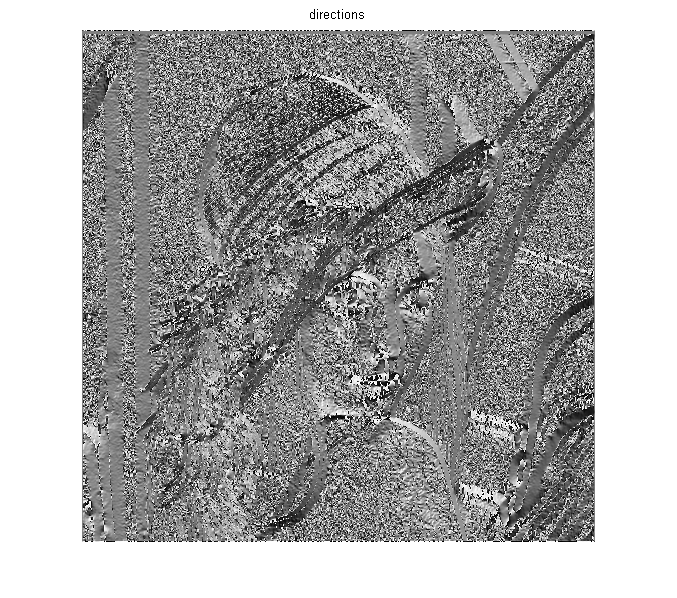
Hard Threshold - Play on the choice of the Threshold T
% Binary Threshold - Play on the choice of the Threshold T T = 5 * median(Norm_Grad(:)); % or choose the treshold using Otzu method (which is however meant for grayscale images, that are % assumed to contain two classes of pixels, thus that their intensity follow a bi-modal histogram) % [T , eff] = graythresh(Norm_Grad(:)); % arbitrary set the threshold % T = quantile(Norm_Grad(:), 0.9); % 0.9 percentile % compute the "gradient mask" by thresholding gradient magnitude mask = Norm_Grad > T; figure(4), imshow(mask), title('gradient mask') % compute the histogram of gradient norm intensities [hh , hh_bins] = hist(Norm_Grad(:) , 1000); % show the histogram with the tresholded value figure(7), bar(hh_bins , hh) hold on plot([T , T] , [min(hh) , max(hh)] , 'g' ,'LineWidth' , 3) hold off % perform Hard Tresholding using a mask Norm_Grad_Binary = double(Norm_Grad > T); Norm_Grad_Hard=Norm_Grad .* Norm_Grad_Binary; % show Gradient Norm figure(2),imshow(Norm_Grad,[]),title('Gradient norm'); % show the mask figure(3),imshow(Norm_Grad_Binary,[]),title('Binary Thresholded gradient norm'); % show HT results figure(4),imshow(Norm_Grad_Hard,[]),title(' Hard Thresholded gradient norm'); % show gradient directions in areas where gradient norm is large (i.e. over the gradient mask) figure(5),imshow(Norm_Grad_Binary.*Dir_Grad,[])
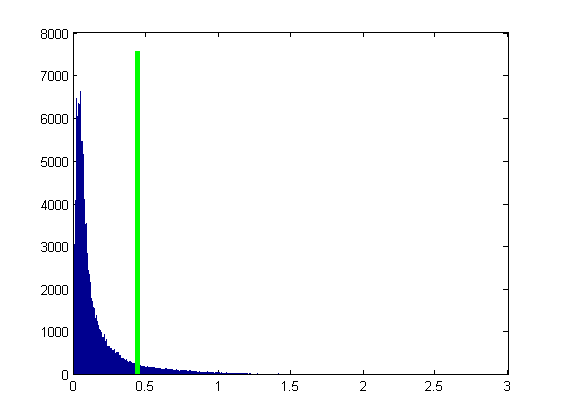
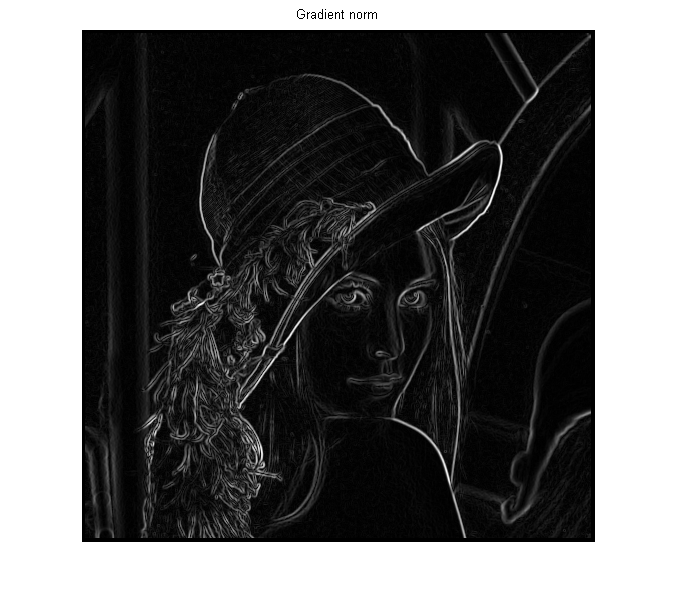
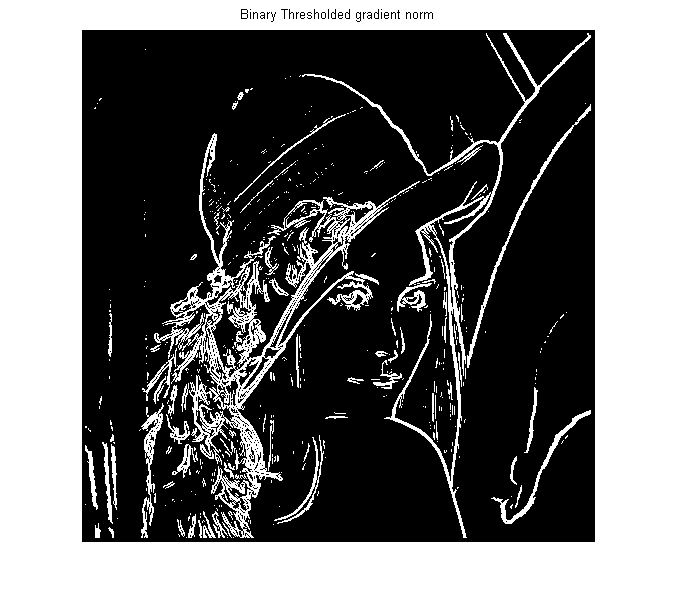
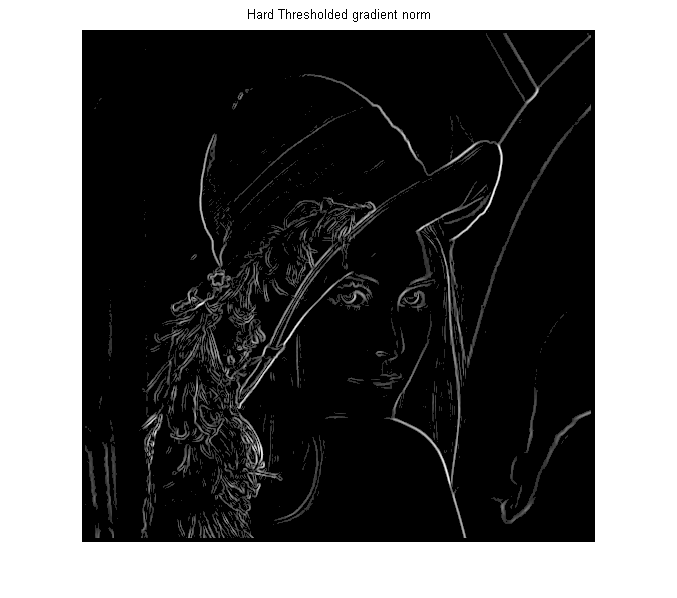
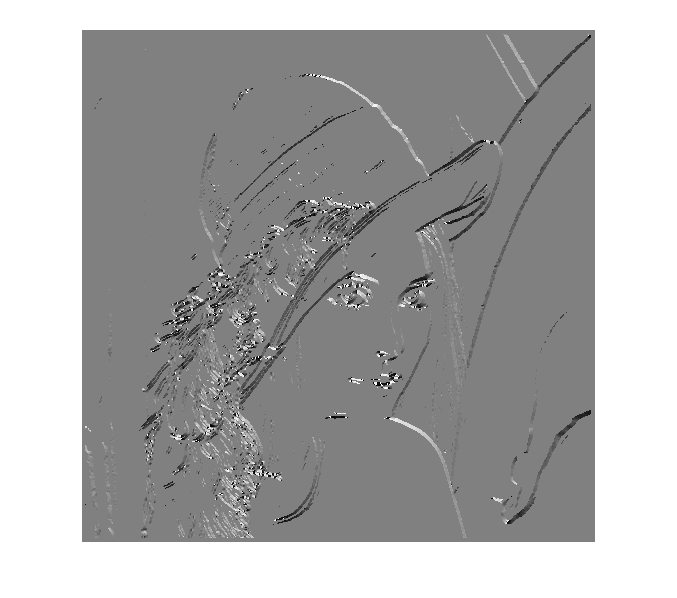
morphological operations on the mask
% dilate via convolution % Norm_Grad_mask=double(conv2(Norm_Grad_Binary,ones(3),'same')>0); Norm_Grad_mask = imerode(Norm_Grad_Binary , ones(3)); Norm_Grad_mask = imdilate(Norm_Grad_mask , ones(5)); figure(3),imshow(Norm_Grad_mask),title('Mask on gradient norm'); % show directions on the dilated mask figure(4),imshow(Dir_Grad.*Norm_Grad_mask,[]),title('Gradient direction'),impixelinfo;
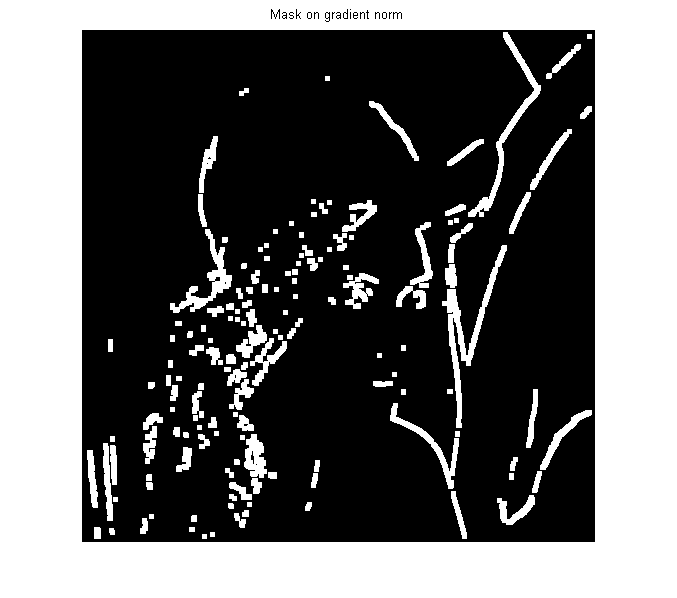
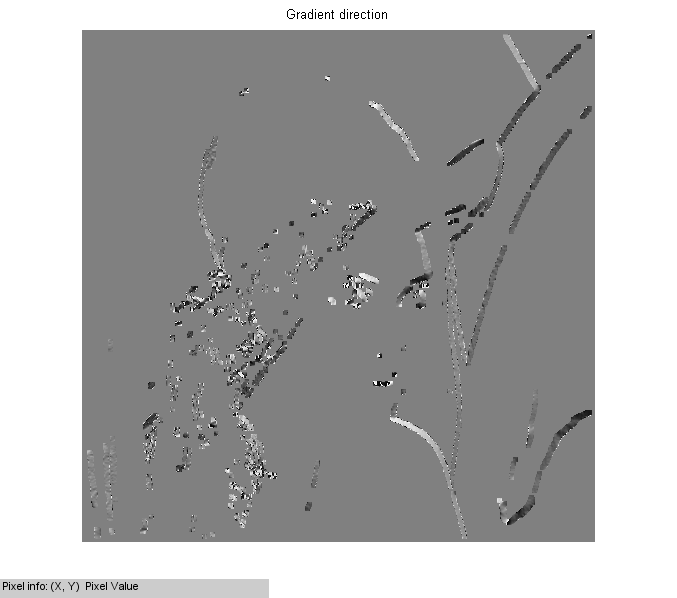
compute edges using Canny method (it includes nonmax suppression and histeresis thresholding)
edgs = edge(y, 'canny');
figure(6), imshow([y, edgs])
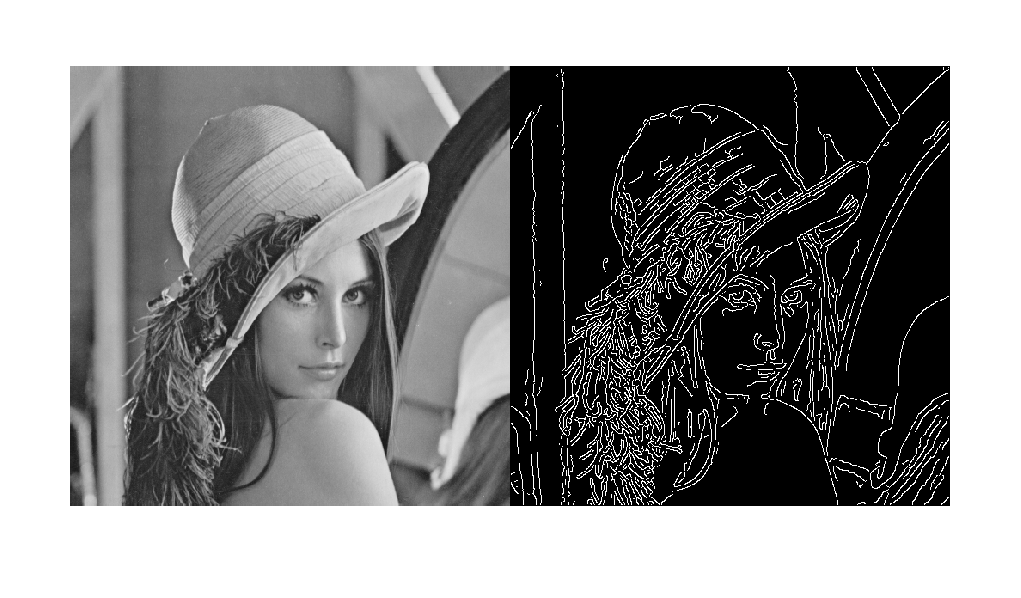